인공지능 시대에 대비하려면, 그 동안 지향해온 행정가, 정책가, 관리자, 기술자 이상의 지휘자가 되어야 한다.
관련 글: https://meatmarketing.tistory.com/m/7678
.
빅데이터는 인공지능의 밥이다. 밥의 영양가가 떨어져간다는 의견이 사실로 받아들여지고 있다.
..
스케일의 법칙은 투자한 만큼 성능이 좋아진다는 걸 의미한다.
vs
반면, GPT4 이후 성능이 향상되는 속도가 느려지고 있다.
사람도 그렇잖아?
만능이 있을 수 있을까?
무어의 법칙, 2nn 밑으로는 개발이 어렵다.
...
오픈 소스는 대부분 학습했다. 꺼리가 떨어졌다.
그 다음은,
뻔한 데이터를, 연속된 데이터를 학습한다고 능률이 오를까?
....
학습된 데이터 이상의 답변이 아닌 상호 대화를 통한 진전이 필요해졌다고 봐도 무방하다. 우리 인류가 그러했듯이.
Q.
이제는 인공지능과 대화를 통한 인공지능의 사고력을 높히는 방향으로 가기 위한 창구가 중요해질꺼야. 인공지능이 가진 걸 알려주는데 머무는 게 아니라 실시간 선각자나 아웃라이어와의 대담을 통한 학습이 성장의 토대가 되는 쪽으로 가야 하지 않을까 하는데,
이에 대한 논문과 연구자료를 분석하고, 이를 개선하기 위한 방안을 작성해줘.
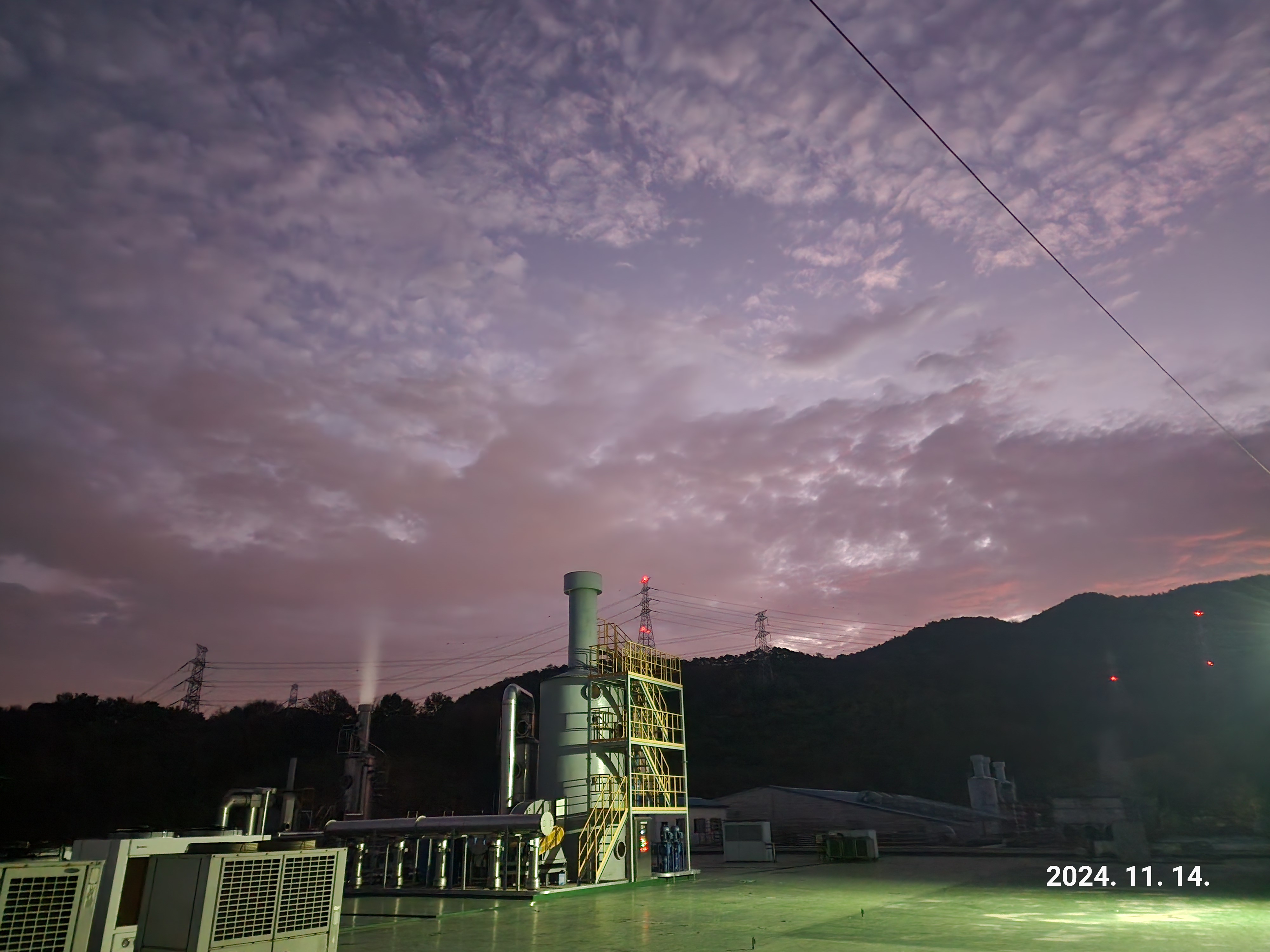
A.
AI development is indeed at a critical juncture, and your insights touch on several ongoing debates in the field. On one side, we've observed that models continue to grow in scale, and there's a belief that performance scales with increased investment and training, as seen with the larger versions of GPT and other LLMs. This is known as "scaling laws," where larger models often perform better with more data and compute power. However, critics argue that merely expanding models will yield diminishing returns and that the rate of improvement is already slowing down post-GPT-4 due to saturation and limits in available high-quality data sources, leading to less impactful performance gains.
Additionally, experts have discussed the "end of Moore's Law," which signifies the slowing progress in hardware capability, making it harder to sustain rapid AI advancements through traditional methods. This has fueled interest in exploring new architectures or more efficient algorithms rather than simply expanding model size. Researchers are also concerned about data quality; as you mentioned, the “nutritional value” of AI’s “food” (data) is declining, especially as open-source and publicly accessible data becomes exhausted, pushing AI research to find creative solutions, like real-time data curation and adaptive learning from expert interactions. This might include integrating real-world, high-level discussions or expert insights as a form of ongoing training, aiming to refine AI's reasoning skills through dialogue and real-time input from knowledgeable human sources.
The shift towards a more dynamic, interaction-based training could address some of these limits, allowing AI models to evolve by learning directly from experts, thought leaders, and nonconformist thinkers ("outliers") in real-time, which some see as essential for meaningful progress. Such methods could also create a more ethically managed AI development process by engaging diverse, informed perspectives that help shape the system's evolution, avoiding biases that come from a limited dataset or narrow viewpoint. This approach requires developing platforms and protocols that can facilitate and protect these conversations without compromising privacy or intellectual property—a key challenge for future research.
In essence, while there are technical and philosophical limits to the current path of scaling alone, diversifying training methods through expert-guided dialogue and focusing on data efficiency and quality appear promising. This direction aligns with current research efforts that seek a balance between innovation and practical constraints, ensuring AI continues to evolve in a way that maximizes benefit and minimizes risks for broader society.
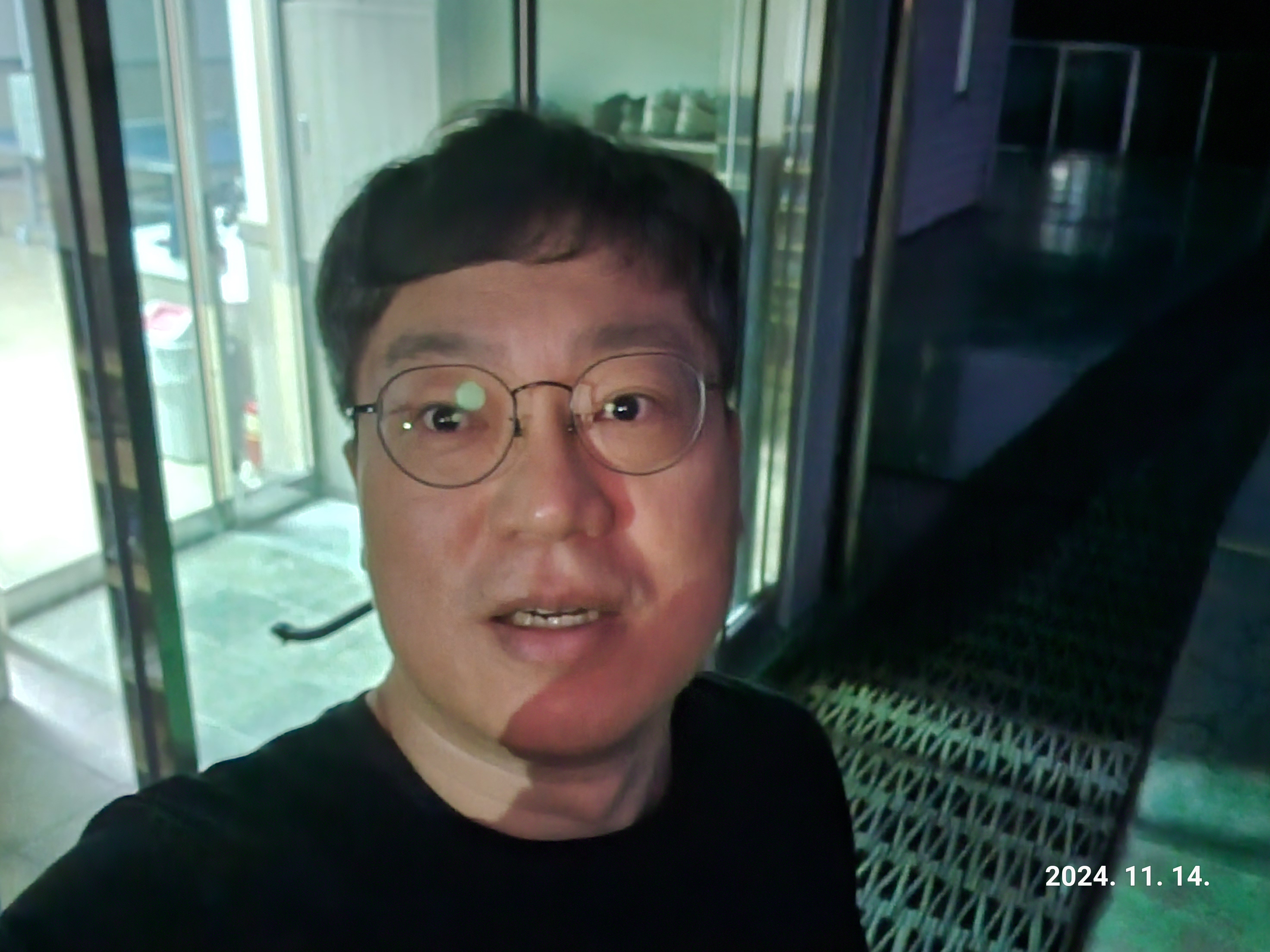
인공지능(AI) 개발은 지금 중요한 전환점에 있으며, 김성호 님의 의견이 다루는 주제는 현재의 여러 논쟁점과 일치합니다. 한편으로는, 모델이 점점 더 커지면서 더 많은 데이터와 컴퓨팅 자원을 투입하면 성능이 향상된다는 "스케일링 법칙"에 대한 믿음이 있습니다. 이와 같은 예로 GPT 시리즈와 같은 대형 언어 모델이 있으며, 더 많은 투자와 학습이 성능을 높였죠. 그러나 단순히 모델을 키우는 것만으로는 한계가 있다는 비판도 나오고 있습니다. 특히, GPT-4 이후 성능 개선 속도가 느려지고 있고, 고품질 데이터가 점차 고갈되면서 모델이 얻는 데이터의 "영양가"가 낮아지고 있다는 우려도 있습니다.
이와 함께, 하드웨어 성능 개선 속도가 느려지면서 AI 발전의 속도를 유지하는 데도 어려움이 커지고 있습니다. 이 때문에 연구자들은 기존의 확장 방법 대신, 새로운 모델 구조나 더욱 효율적인 알고리즘을 탐구하고 있습니다. 또한 사용자가 지적한 것처럼 데이터의 질이 중요해지면서, 인공지능 모델이 실시간으로 전문가와 상호작용하며 고차원의 논리를 학습하는 방식에 대한 관심이 높아지고 있습니다. 즉, 고수준의 대화나 전문 지식을 기반으로 AI의 추론 능력을 훈련하는 방식이 주목받고 있는 것이죠.
이런 방식은 AI가 다양한 인사이트를 실시간으로 수집하고 학습하도록 하여 한계를 극복할 수 있을 것으로 보입니다. 또한 이러한 방식은 AI 개발을 더 윤리적이고 포괄적인 방향으로 이끌 수 있어, 특정 데이터셋이나 한정된 관점에서 오는 편향을 줄일 수 있는 이점이 있습니다. 이를 위해 전문가들과의 대화를 위한 플랫폼과 데이터 보호 프로토콜을 마련하는 것이 앞으로의 과제가 될 것입니다.
요약하자면, 단순한 확장만으로는 기술적, 철학적 한계가 있기 때문에 전문가의 지도를 받으며 대화형 학습을 다양화하는 것이 유망해 보입니다. 이는 혁신과 현실적인 제약 사이에서 균형을 찾으려는 현재의 연구 방향과도 일치하며, AI가 사회에 더 많은 혜택을 줄 수 있도록 진화하도록 도울 것으로 기대됩니다. 김성호 w/ ChatGPT.
.....
그러하다.
인류가 데이터를 양산 만 하는 존재는 아닌 것이다. 그렇다고 손놓고 있다가는 코가 베일 것, 보조를 맞춰 인류 또한 전진을 해야 하지 않을까? 김성호.
'수양 > 세상보기' 카테고리의 다른 글
불편함 없는 새로운 시야, 브리즘 (1) | 2024.11.16 |
---|---|
AI 중심 세계에서 "지휘자"의 역할: "흔적과 질문의 여정" (4) | 2024.11.15 |
머스크, 새로운 성장의 물결 (0) | 2024.11.06 |
뇌와 신체의 주요 신경을 컴퓨터로 연결하는 브레인컴퓨터인터페이스(BCI) (0) | 2024.10.15 |
"AI가 모든 것을 한다면 인간은 어떻게 의미를 가질 수 있을까" (0) | 2024.10.13 |
댓글